t-intervals vs. z-intervals
모평균(population mean)을 추정할 때 모평균과 모분산을 모두 모를때(both unknown) t-procedure를 이용한다.
정리하자면 다음과 같은 상황에서 모평균을 추정할 때 t-procedure를 사용한다.
- population이 normal distribution임이 알려져 있다. (매우 강력한 가정!)
와 가 알려져 있지 않다. (both unknown)- sample size
은 사실 상관이 없다.
※ CLT에 의해
※
Remark: Normal related statistics
확률변수
와 는 독립이다.
Introduction
모평균을 추정할 때 점추정이 좋은가 구간추정이 좋은가?\
예1)
MLE에 의해
그렇다면
예2)
이렇듯 모평균을 추정할때 "모평균이 어떤 값일 확률"과 같은 표현은 적절하지 못하다.
대신에, "모평균이 어떤 구간에 있을 확률"과 같은 표현(
-Confidence Interval
이제
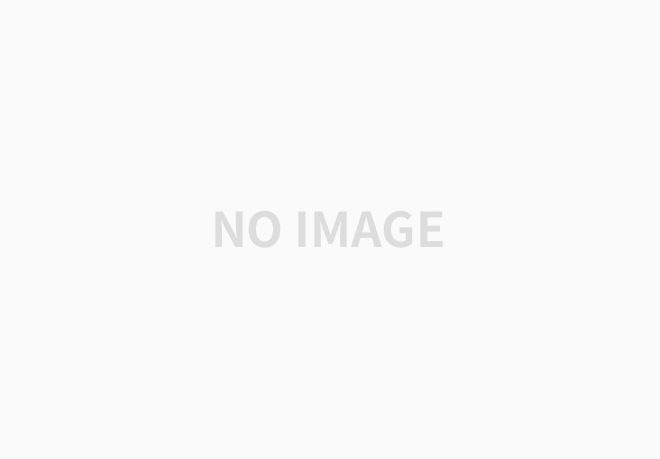
위
우리가 얻은 데이터(observed/obtained values, data)에 따라 위 구간은 달라진다. 즉
이때
Confidence Length
Confidence Length
Effect of Sample Size
confidence length에서 우리는 특정 길이를 만족하는
(같은
confidence length가
Additional Sampling
이미 먼저 pilot study로
근데 신뢰구간을 줄이기 위해(
을 계산하고, 추가로 뽑을 샘플 수는
Example
첫번째 샘플 크기가
전체 샘플 크기는
따라서 전체 샘플 크기는
Simulation and Confidence Interval
신뢰구간을 계산할 때, 우리는 주어진 데이터가 한번뿐이다. 그래서 95%의 신뢰구간이 와닿지 않을 수 있다.
만약에
모평균은 unknown일 뿐이지 어딘가에 고정된 값을 가지므로 다음과 같다.
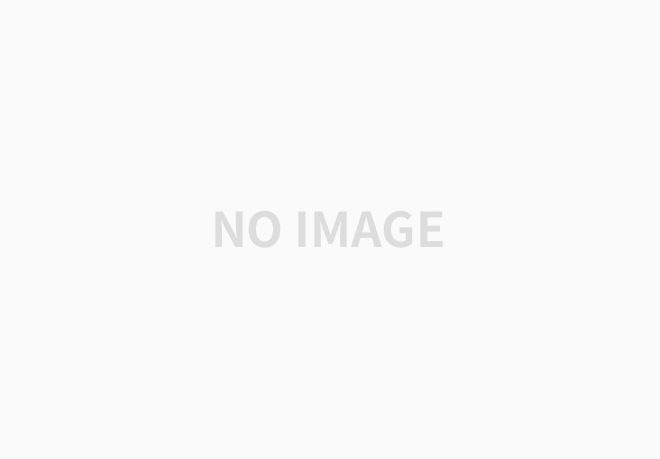
Some Notations
확률변수는 대문자로, 실제 관측값은 소문자로 표기한다. 아래 tricky notation으로 살펴보면 다음과 같다.
(1)
(2)
(3)
따라서 위 확률은 True라면
(4)
One-Sided Confidence Intervals
다음 두개의 사실을 remind하자
따라서 confidence level이
비슷하게, lower bound에 대한 confidence interval은
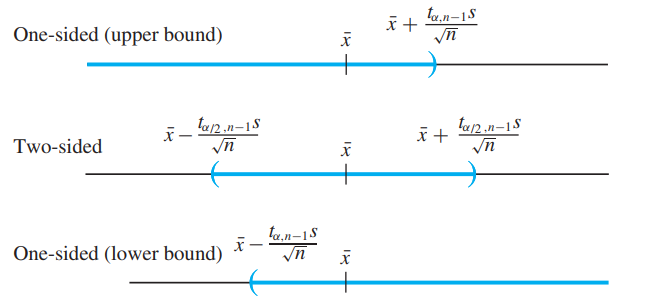
※
※ one-sided interval에서는 신뢰구간의 길이를 정의하지 않는다. upper/lower bound에 관심이 있는 경우이므로 confidence interval length는 고려대상이 아니다.
※ two-sided interval은
※ one-sided lower confidence interval은 upper bound를, one-sided upper confidence는 lower bound를 제공한다.
-intervals
다음의 경우에는
- population distribution이 정확히 normal이고
- 모평균
는 알려져 있지 않지만 모분산 는 알려져 있을 때
※ sample size
※ population distribution이 normal이면, 정확한 confidence interval을 구한다. (exact coverage, not approximation)
다음의 사실을 이용한다.
따라서 two-sided confidence interval은 다음과 같다,
one-sided interval은 다음과 같다.
Summary
- 데이터가 normality를 만족할 때,
가 known이면 z-procedure 가 unknown이면 t-procedure
- 데이터가 normality를 만족하지 않을 때,
- sample size
이 충분히 크면 z-procedure (이때 정확한 coverage가 아니라 approximation이다)
- sample size
- 충분히 큰 sample size는 이론상 20~30이지만, 통계학과 교수님께서는 실제로는 50 이상은 되어야 한다고 한다.