Lazy vs Eager Learning
Lazy Learning (e.g. instance-based learining): training data를 저장하고 new data(test data)를 입력받을 때 classifiy한다.
Eager Learning: new data(test data)를 입력받기전에 classification model을 먼저 구성한다.
이전에 다룬 decision tree, naive bayes classifier는 eager learning이다.
Efficiency
Lazy learning은 training time은 짧지만 predicting에서 더 많은 시간이 걸린다.
Accuracy
Lazy Learning은 더 복잡한 결정 공간(more complex decision space)을 구성할 수 있다.
Eager Learning은 다소 단순한 가정(single hypothesis)로 모든 instance space를 다룬다.
Instance-based learning
학습데이터를 저장하고, 새로운 데이터가 들어오기 전까지 분류하지 않는다
k-nearest neighbor approach, Case-based reasoning 등이 대표적인 instance-based learning이다.
Nearest Neighbor Classifiers
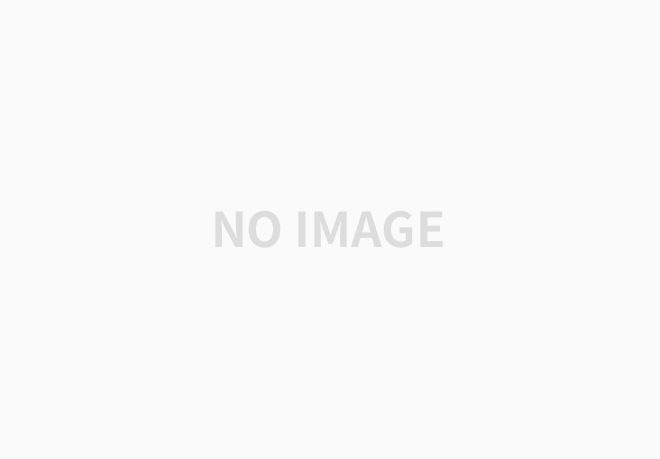
Input
미리 저장된 레코드 집합 (a set of stored records)
레코드 끼리의 거리를 계산할 지표(distance metric)
가까운 이웃 레코드의 개수
Prediction
다른 학습 레코드와의 거리 계산
최근접이웃들의 class label을 이용하여 unknown record의 clas label 예측(e.g. majority vote)
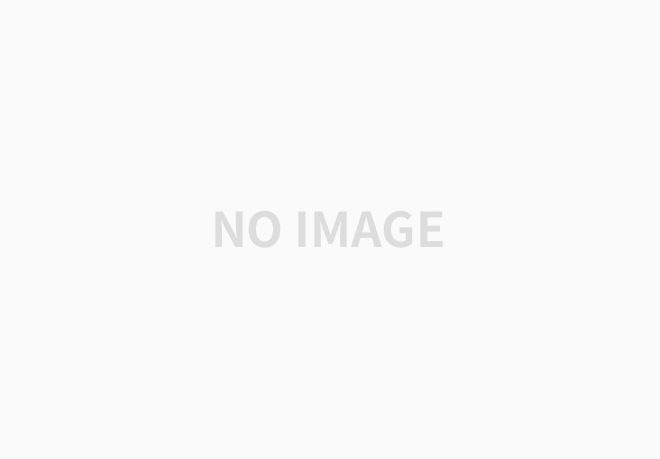
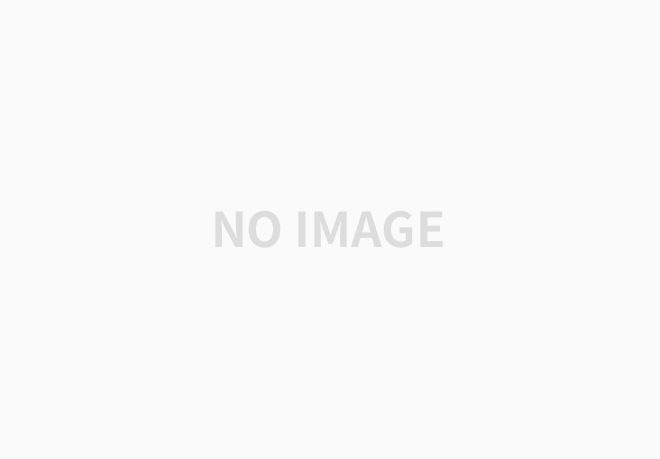
Issues
kNN classifier는 크게 2가지 문제를 갖는다. 주로
The value of
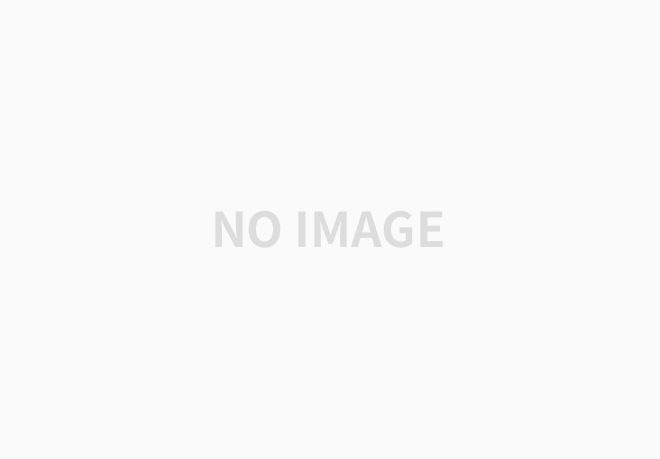
Scaling and High-dimensional data
distance를 계산하기 때문에 수치의 크기에 영향을 주기 때문에 attribute들은 반드시 스케일링되어야 한다.
그렇지 않으면 크기가 가장 큰(dominant) 속성에 영향을 받는다.
예를 들어, 데이터가 (사람의 키, 사람의 몸무게, 평균 연소득)이라면, 연소득의 단위가 가장 크기 때문에 키나 몸무게는 distance 내에서 무시되어 classify될때 아무런 영향을 주지 못한다.
그리고 데이터 차원이 너무 크면, 직관에 반하는 결과(counter-intuitive result)를 얻을 수 있다.
'스터디 > 데이터사이언스' 카테고리의 다른 글
[CS246] Spark: Extends MapReduce (0) | 2023.09.09 |
---|---|
[CS246] MapReduce (0) | 2023.09.08 |
[Data Science] Mediator, Moderator (0) | 2023.05.15 |
Dummy coding, Effect Coding (0) | 2023.05.15 |
[Data Science] Logistic Regression (0) | 2023.05.14 |